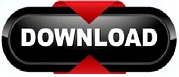
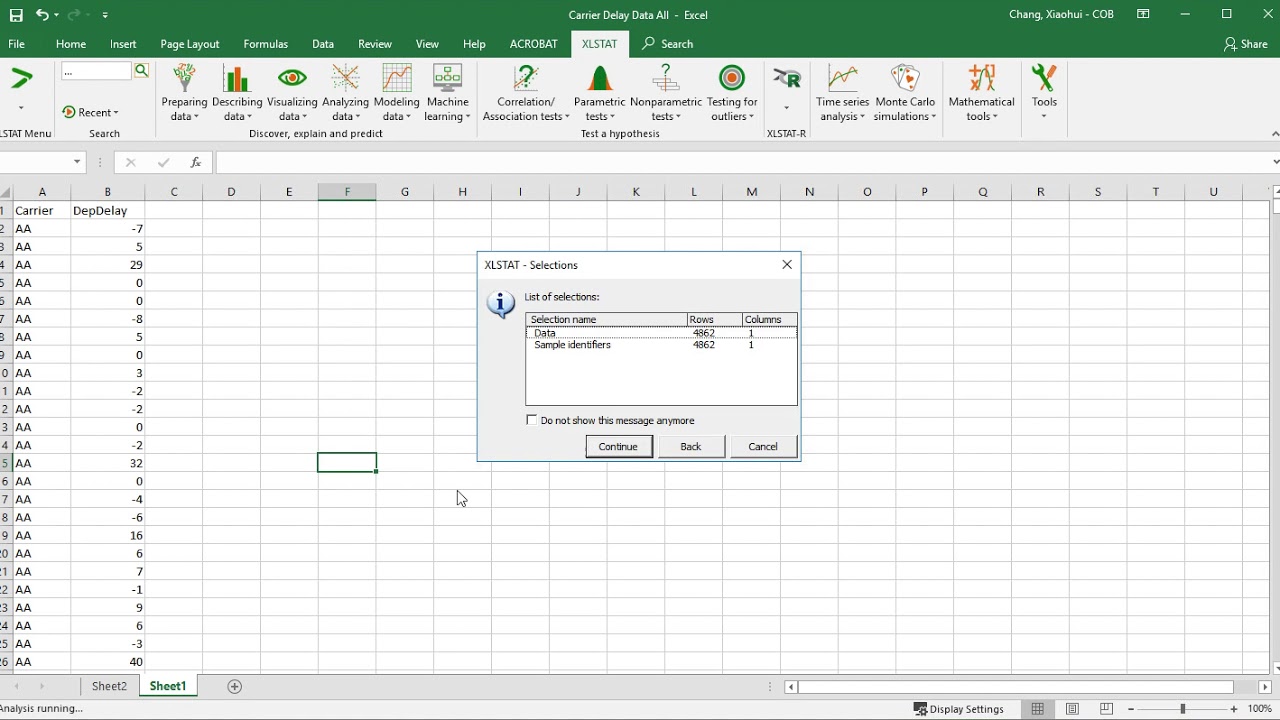
If the data are normally distributed, the result would be a straight diagonal line ( 2). The actual z-scores are plotted against the expected z-scores. The scores are then themselves converted to z-scores. This is the expected value that the score should have in a normal distribution. After data are ranked and sorted, the corresponding z-score is calculated for each rank as follows: z = x - ᵪ̅ / s. The P-P plot plots the cumulative probability of a variable against the cumulative probability of a particular distribution (e.g., normal distribution). The stem-and-leaf plot is a method similar to the histogram, although it retains information about the actual data values ( 8). The frequency distribution that plots the observed values against their frequency, provides both a visual judgment about whether the distribution is bell shaped and insights about gaps in the data and outliers outlying values ( 10). The frequency distribution (histogram), stem-and-leaf plot, boxplot, P-P plot (probability-probability plot), and Q-Q plot (quantile-quantile plot) are used for checking normality visually ( 2).

However, when data are presented visually, readers of an article can judge the distribution assumption by themselves ( 9).

Visual inspection of the distribution may be used for assessing normality, although this approach is usually unreliable and does not guarantee that the distribution is normal ( 2, 3, 7). The purpose of this report is to overview the procedures for checking normality in statistical analysis using SPSS. It is important to ascertain whether data show a serious deviation from normality ( 8). Although true normality is considered to be a myth ( 8), we can look for normality visually by using normal plots ( 2, 3) or by significance tests, that is, comparing the sample distribution to a normal one ( 2, 3). According to the central limit theorem, (a) if the sample data are approximately normal then the sampling distribution too will be normal (b) in large samples (> 30 or 40), the sampling distribution tends to be normal, regardless of the shape of the data ( 2, 8) and (c) means of random samples from any distribution will themselves have normal distribution ( 3). If we have samples consisting of hundreds of observations, we can ignore the distribution of the data ( 3). With large enough sample sizes (> 30 or 40), the violation of the normality assumption should not cause major problems ( 4) this implies that we can use parametric procedures even when the data are not normally distributed ( 8). Normality and other assumptions should be taken seriously, for when these assumptions do not hold, it is impossible to draw accurate and reliable conclusions about reality ( 2, 7). The assumption of normality is especially critical when constructing reference intervals for variables ( 6). Many of the statistical procedures including correlation, regression, t tests, and analysis of variance, namely parametric tests, are based on the assumption that the data follows a normal distribution or a Gaussian distribution (after Johann Karl Gauss, 1777–1855) that is, it is assumed that the populations from which the samples are taken are normally distributed ( 2- 5). Statistical errors are common in scientific literature, and about 50% of the published articles have at least one error ( 1).
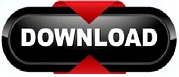